Have you heard anyone mention AI and Machine Learning in one sentence? It's easy to ask whether they're related. Although they might sound similar, they're not twins; there are vital differences between them that you should know about! Don't worry, though; we are here to explain everything!
What is Artificial Intelligence?
Artificial Intelligence (AI) is the ultimate dream for superintelligent machines—machines that can learn, solve problems, and make decisions independently without needing humans for guidance or instruction. That means creating machines that only sometimes depend on humans telling them what to do next!
Big names in AI companies, such as Google, DeepMind, and IBM, are at the forefront of AI research and are investing heavily in the development of more intelligent machines. From self-driving cars to facial recognition on your phone to Siri's ability to assist with tasks to special tools used by doctors to diagnose diseases, AI is all around us, making life simpler and cooler in countless ways.
What is Machine Learning?
Machine Learning (ML) is a vitally important AI tool. Think of it like this: machines are learning automatically from data, just like we do with the help of Machine Learning! They can identify patterns and predict outcomes without needing specific instructions from someone constantly telling them what to do! And perhaps the coolest part? They do all this without someone telling them what they should be doing! For instance, an ML system could use your email messages to identify spam or use your music preferences to recommend new songs to you.
How Does Machine Learning Work?
Machine learning, or ML for short, is all about teaching computers to learn from data to make smart predictions or decisions on their own. It's similar to teaching a student: it goes through a series of steps until it becomes "knowledgeable" about its tasks. Here’s the breakdown:
- Data Preparation and Collection: Just as students need textbooks, a good ML model learns best from quality data. This could include photos, numbers, text—anything relevant to the model's task. Before use, the data needs cleaning: errors, duplicates, and unnecessary details are removed to ensure the model studies only high-quality information.
- Choosing an Algorithm: Algorithms represent the model's "thought process," acting as guidelines or patterns for processing data. For instance, CNNs are used in image tasks because they excel at recognizing visual patterns, while regression models handle predictions based on prior trends. The task’s nature determines which algorithm is used.
- Training The Model: Training resembles solving practice questions. The model processes the data and continuously adjusts to learn the relationships within. Each round improves its ability to identify patterns, increasing its accuracy at the given task.
- Testing and Refining: Once trained, the model is tested with new data, like taking a quiz to assess its learning. If needed, developers fine-tune the model to boost its accuracy. This step ensures the model can perform well in real-world situations.
- Making Predictions and Adapting with Feedback: The model then goes live, making predictions or decisions in real time. Over time, it adapts based on new data or feedback to stay relevant and accurate. For example, a streaming service’s ML model may start recommending songs based on your listening habits, improving as you like or skip tracks.
Types of Machine Learning Approaches
Different ML approaches suit various tasks. Here’s a quick overview of the main types:
- Supervised Learning: This approach resembles learning with a teacher who provides answers. The model learns from labeled data, where each segment has a known output. A common example is email spam filters, which use supervised learning with emails labeled as spam or not spam, allowing the model to detect patterns.
- Unsupervised Learning: Here, the model learns from data independently, looking for patterns or clusters. It’s often used in data segmentation, such as identifying customer segments based on purchasing habits.
- Semi-Supervised Learning: This method uses both labeled and unlabeled data, which is useful in fields like healthcare where labeled data is limited. The model learns from a small labeled set and applies its understanding to the larger, unlabeled set.
- Reinforcement Learning: In this approach, the model learns by trial and error, receiving feedback for its actions. It’s commonly used in robotics, where the model learns optimal actions through rewards and penalties.
How AI is Transforming Businesses
AI companies are turning to machine learning (ML) techniques to streamline operations, enhance customer experiences, and increase revenue. One such AI company, Waymo (a subsidiary of Google), is developing autonomous vehicles equipped with self-driving technology and robot taxis that will eventually operate across multiple cities; extensive testing will generate an immense amount of data that will allow AI systems to refine themselves over time and increase safety, efficiency, and mobility.
Rubrik, a multi-cloud data control company, employs AI to continuously assess large volumes of telemetry and metadata collected on its cloud environment in real-time, which allows it to optimize cloud costs, predict outages, and identify security vulnerabilities - as well as drive significant growth rates for itself and its clients—Fortune 500 organizations, government agencies, and significant universities among them are its clients.
1. AI vs. Machine Learning: What's the Difference?
Here's the key difference between AI and Machine Learning: AI acts like the chef who wants to prepare a fantastic meal; Machine Learning serves as its recipe book with detailed instructions for reaching that goal. Together, they work as one. AI establishes its overall plan, while ML provides learning muscle to make that plan come to fruition!
2. Unveiling the Power of AI and Machine Learning in Everyday Life
Look around! AI and Machine Learning (ML) may already be part of your everyday life. From alarm clocks that use ML to learn your sleep patterns to social media algorithms that curate personalized news feeds with relevant content, AI/ML is everywhere!
Your favorite cup of coffee brews perfectly, thanks to AI-powered factory machines that adjust settings according to bean type and weather. When heading out for the day, traffic navigation apps utilize real-time data analysis and machine learning (ML) techniques to suggest the fastest route, avoiding traffic jams and saving you precious time.
These are just a few examples. AI and ML are transforming the way we live, from entertainment to health care to security and transportation. As these fields grow, we can anticipate greater applications that help make our lives easier, safer, and more enjoyable.
3. AI and Machine Learning: Working Together for a Smarter Future
Imagine the possibilities that unfold when Artificial Intelligence (AI) and Machine Learning (ML) combine forces. From doctors studying medical scans with greater precision to autonomous cars navigating city streets, these technologies are shaping a smarter future.
AI can create ideas and visions, while machine learning provides the ability to learn and adapt. Together, they have the potential to transform entire industries, including:
- Renewable energy production: AI and ML can optimize energy distribution and improve the efficiency of renewable energy sources.
- Climate change mitigation: AI/ML development companies can use AI and ML to predict climate patterns and develop sustainable solutions for reducing emissions.
- Space exploration: These technologies assist in analyzing vast amounts of space data, leading to new discoveries and insights.
- Healthcare: AI development services are enabling faster, more accurate diagnoses, while ML tailors personalized treatment plans for patients.
- Autonomous vehicles: ML algorithms enable self-driving cars to safely navigate roads, enhancing safety and convenience.
However, as these technologies evolve, it’s essential to remember that power comes with great responsibility. AI and ML should be employed with caution and ethical considerations. By working closely with engineers, scientists, and policymakers, we can ensure these innovations have positive impacts.
As machine learning (ML) becomes integral to business processes, AI/ML development companies must focus on:
- Upholding ethics and transparency in all AI development services to build trust with users.
- Avoiding misleading or biased models that could harm the company’s reputation or incur regulatory fines.
- Ensuring that algorithms are fair and transparent, so users can trust the results.
Ethical Dimensions of AI and Machine Learning
As AI and ML technologies expand, it’s essential for an AI development company to address ethical concerns like fairness, privacy, and accountability. Ensuring these technologies benefit everyone equitably is key to a responsible and sustainable future for machine learning services.
1. Fairness and Bias
AI decisions reflect the data they’re trained on. Biased data leads to biased outcomes, particularly in law enforcement, hiring, and healthcare. Developers must focus on diverse datasets, proactive bias detection, and inclusivity to ensure fair outcomes and eliminate harmful stereotypes.
2. Privacy and Data Security
AI heavily relies on personal data, and this raises major concerns about privacy. 60% of businesses in 2023 experienced AI-related data breaches, and this underlines the necessity to impose tight security. Transparency, proper handling, and compliance with laws such as GDPR are essential to maintaining the privacy of the individual and establishing trust among users.
3. Accountability in AI Systems
AI’s autonomous decisions could have life-changing implications. Transparent accountability is required, most urgently in healthcare, whose mistakes could be lethal. Transparencies in decision-making by the creators and the organizations are required to retain control by humans to maintain responsibility and to cover up any mistakes.
4. Transparency in AI Decisions
To foster trust, the AI systems also have to be explainable. The users must be shown the reasons the decisions are so complex. The explainable AI shall give assurance, reinforce users' confidence, and also reinforce compliance in industries like healthcare and finance, which are extremely sensitive.
5. Environmental Impacts
The environmental influence of training the models in AI grows, requiring them to use huge processing power. It's the duty of the developers to provide energy efficiency and the use of green sources to rein in the carbon footprint of the use of AI.
Emerging Trends in AI and Machine Learning
In that direction, the future of AI and ML will head toward systems that are more transparent, adaptive, and proficient in solving very complex global challenges.
- Explainable AI: There is an ever-increasing demand both by users and regulators that there be more transparency in AI-driven decisions, particularly in applications that are very critical to life, such as healthcare. Explainable AI refers to systems capable of delivering understandable explanations as to the reason for their conclusions. This will be particularly important to earn trust and comply with regulations.
- Advanced Automation: AI is evolving its capabilities beyond simple and repetitive tasks towards more complex tasks, which are driving innovation in areas such as logistics, customer service, and even creative domains. This is likely to streamline operations and unlock new possibilities in the respective industries as it is happening.
- Climate Action: Artificial intelligence promises to help in several ways in facilitating actions on climate change through efficient resource utilization, assisting in conservation projects, and providing data-driven insights for developing truly working sustainable outcomes in all endeavors. In the face of growing climate challenges, the footprint of AI in this area is likely to increase.
- Personalization Technology: It is one wherein continuous refinement of users' experiences is performed with the help of AI-driven applications in the fields of health to retail. AI makes user experiences engaging, responsive, and more relevant by personalizing interactions based on the preference of an individual.
- Cybersecurity: Gradually growing cyber threats have placed AI as an essential tool in the identification and response process of digital risks. Capable of detecting in real time any threats that might occur, it modifies the defenses as necessary, turning it into an indispensable ally for the protection of digital environments.
These trends are indicative of the capability that AI has not only to change industries but also to handle very complex challenges on the road to a more intelligent and adaptive future.
Conclusion
Artificial intelligence and machine learning promise an exciting future! As these technologies advance, their potential impact could spread from healthcare and transportation to entertainment and education—so be prepared when someone mentions AI/ML! Understanding AI/ML technologies is a great place to start for anyone in IT or curious about future web and mobile app development; keep an eye out for these intelligent systems—they may power the next big thing you use daily!
FREQUENTLY ASKED QUESTIONS (FAQs)
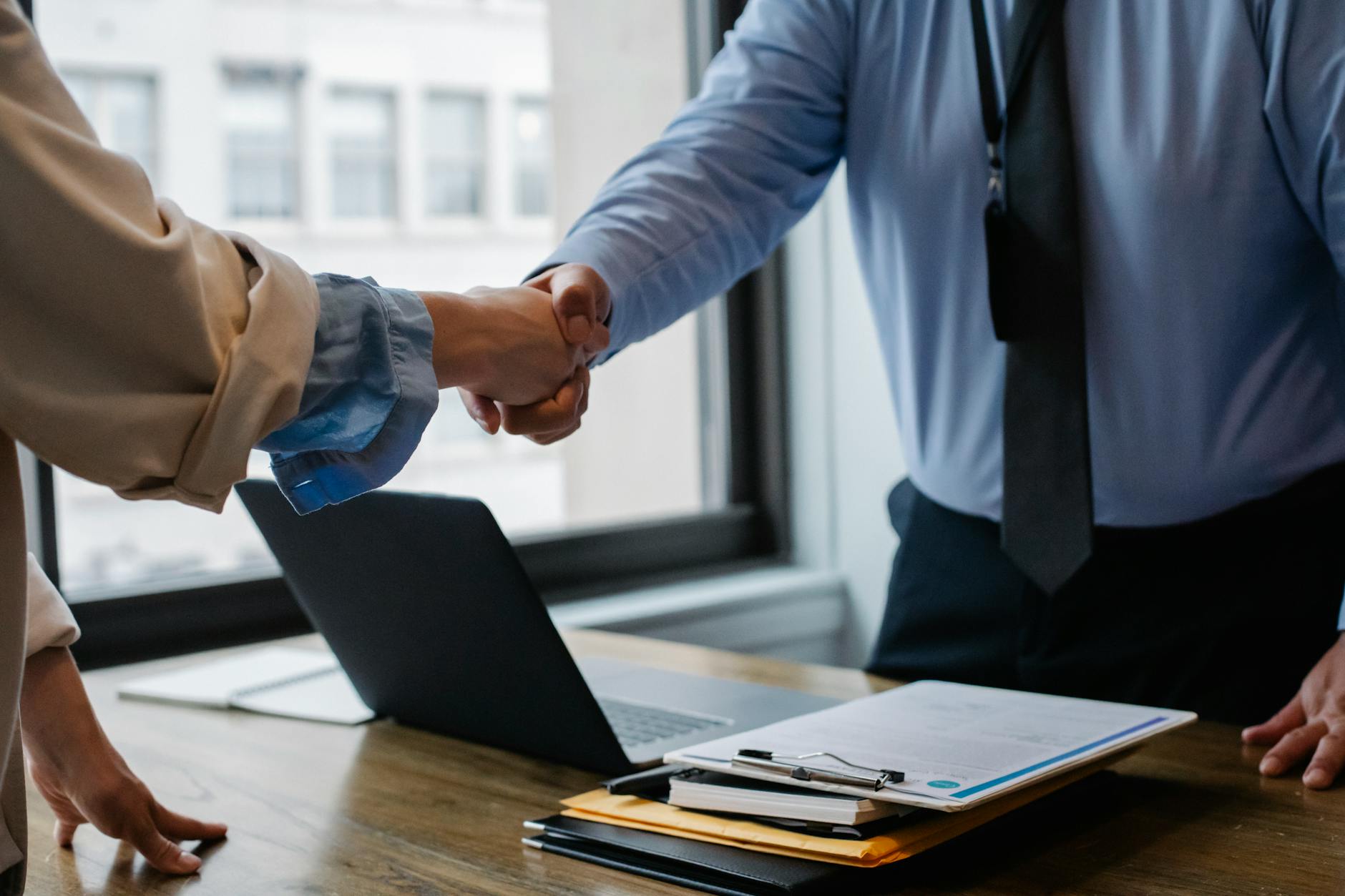