Smart business decisions are being built more and more on advanced data analytics. Collecting data is only one aspect; translating raw data into real-time insights that enable companies to expand, change, and beat their rivals is what really counts. The correct analytical method can simplify operations and open new possibilities, whether you work in banking, healthcare, or retail.
IDC really forecasts that by 2025, worldwide data and analytics expenditure will top $300 billion. Such an investment demonstrates how much businesses value data. Traditional analytical techniques simply don't cut it anymore with changing technology and growing consumer expectations. Dashboards are insufficient for companies; they also want predictive power, automated insights, and action-ready plans.
This blog will clarify what advanced data analytics truly means, the leading methodologies and tools being utilized in 2025, and how companies, just like yours, can use them for improved decision-making, enhanced results, and a significant edge in a competitive environment.
What is Advanced Data Analytics?
Advanced data analytics is the use of sophisticated tools and methodologies to glean more profound insights, trends, and patterns from unanalyzed raw data. Unlike simple analysis approaches that emphasize past data, sophisticated techniques such as predictive modeling, machine learning, and real-time analytics enable companies to foresee outcomes, optimize processes, and personalize customer interactions.
In today's competitive universe, conventional methods of data analysis are not sufficient anymore. To reach from reactive to proactive decision-making, firms are embracing advanced analytics. From identifying market movements ahead of time to optimizing supply chain efficiency, these insights are transforming the way industries operate.
Here are some commonly used advanced data analysis techniques and tools in 2025:
- Predictive Analytics Techniques: Anticipate customer behavior and sales trends with future-oriented insights.
- Machine Learning Models: Use algorithms to analyze large datasets and continuously improve outcomes.
- Real-Time Data Processing: Make informed decisions instantly with streaming analytics from IoT, sensors, or apps.
- Data Visualization Tools: Communicate insights clearly with dynamic dashboards and visual storytelling.
- Text Analytics & NLP: Derive meaning from unstructured data like customer reviews, social media, and support chats.
Large corporations are no longer the only ones using these techniques of data analysis. For companies of all sizes, cloud-based solutions and AI-powered platforms have made sophisticated analytics available.
These methods enable better judgments at every level, whether you are utilizing complex statistical analysis or including data analytics tools and techniques in your digital strategy.
Advanced analytics methods help companies not only to maximize internal operations but also to propel customer-centric innovation and long-term expansion.
Why Businesses Need to Adopt These Techniques in 2025
Advanced data analytics is becoming a corporate need rather than only a competitive advantage. As data generation continues to rise exponentially, companies that lag behind in adopting data analytics methods risk falling behind both market trends and consumer expectations. Evidence-based insights are becoming increasingly necessary to drive agile and informed choices, whether one is researching consumer behavior, forecasting sales, or optimizing supply chain effectiveness.
Indeed, a recent Forbes poll found that 64% of world leaders think data-driven policy is essential for their success. Most companies, however, still use antiquated data analysis techniques that fall short of the need for speed, accuracy, and individualization in modern society.
What causes this? Often, it's a combination of old infrastructure, insufficient internal knowledge, or just not knowing where to begin.
Here’s why businesses should take action now:
- Real-time insights: Advanced analytics tools process vast amounts of data instantly.
- Better decision-making: Predictive analytics reveals what might happen next, before it does.
- Increased efficiency: Automated techniques reduce manual analysis time significantly.
- Personalized customer experiences: Data-driven decisions boost engagement and loyalty.
- Competitive advantage: Stay ahead by responding faster to trends and risks.
These advantages are no longer exclusive to big companies. Even small and mid-sized companies can get actionable intelligence with the availability of scalable data analytics tools and methods.
Being reactive is no longer an option in 2025 and beyond; proactivity supported by data is the new standard. Companies that neglect to include sophisticated analytics run the There is a danger in making expensive choices based on gut feelings instead of informed knowledge. Next, we will examine the most efficient advanced data analytics tools that are transforming the operations of contemporary companies.
Top 7 Advanced Data Analytics Techniques to Know in 2025
1. Regression Analysis
One of the basic techniques of data analysis used to investigate correlations between dependent and independent variables is regression analysis. By means of pattern recognition and consequence prediction, it helps companies to make data-driven choices. Widely used are regression models—especially linear and logistic regression—from sales trends to customer churn forecasts.
Key Highlights:
- Ideal for predictive analytics in finance, sales, and marketing.
- Supports decisions by quantifying the impact of variables.
- Enhances data analytics techniques. It is used through measurable outcomes.
- Used in customer behavior forecasting and ROI modeling.
- This is a core element of advanced data analytics in business strategies.
2. Factor Analysis
A dimensionality reduction method, factor analysis reduces complicated data sets This involves identifying the underlying variables (factors) that influence the data. It's particularly beneficial when dealing with multivariate statistics or big survey datasets.
Key Highlights:
- Reduces data complexity for easier interpretation.
- Helps identify hidden variables that affect multiple metrics.
- Boosts model efficiency in machine learning workflows.
- Often used in psychographic profiling and social sciences.
- Valuable in the data analytics methodology for simplification.
3. Cohort Analysis
A cohort study splits data into parts based on shared traits or timelines, such as consumers who signed up in the same month. Examining behavior patterns throughout time depends on this study.
Key Highlights:
- Tracks user retention, engagement, and churn.
- Frequently used in SaaS, e-commerce, and mobile app analytics.
- Assists in understanding lifecycle behavior.
- Enables personalized marketing and loyalty strategy.
- This is a leading technique of data analysis in research and UX.
4. Monte Carlo Simulation
By modeling thousands of situations with random variables, Monte Carlo simulation helps businesses grasp risk. In sectors with great uncertainty, such as finance, supply chain, and project management, it is especially crucial.
Key Highlights:
- Predicts probable outcomes using stochastic models.
- Enhances strategic planning by quantifying risk exposure.
- Aids in capacity planning and inventory optimization.
- Uncertainty modeling is central to advanced data analytics tools.
- Supports decision-making in long-term capital investment.
5. Cluster Analysis
Based on characteristics, cluster analysis organizes comparable data points into clusters. In market basket analysis, anomaly identification, and client segmentation, this is a fundamental approach.
Key Highlights:
- Popular technique in marketing and fraud detection.
- Improves personalization by uncovering user groupings.
- The system works well with big data analytics techniques.
- Supports unsupervised learning in machine learning applications.
- Valuable in analytical techniques used in big data visualization.
6. Time Series Analysis
Examining trends over time, time series analysis finds seasonal patterns and produces precise projections. For sectors depending on time-based measures like retail, stock markets, and transportation, such analysis is absolutely vital.
Key Highlights:
- Analyzes data collected at regular intervals.
- Ideal for demand forecasting and capacity planning.
- Integrates models like ARIMA, Holt-Winters, and Prophet.
- Helps detect anomalies and automate alerts.
- A must-have in any suite of data analysis tools and techniques.
7. Sentiment Analysis
Using natural language processing (NLP), sentiment analysis identifies the emotional tone underlying textual data. It offers companies knowledge on consumer comments and public opinion.
Key Highlights:
- Extracts emotional context from social media, reviews, and surveys.
- Enhances brand monitoring and reputation management.
- Supports automated classification (positive, neutral, negative).
- Important in customer experience management.
- A growing area in advanced data analytics for CX and PR teams.
As data volumes grow and customer demands evolve, mastering these advanced techniques allows businesses to stay competitive, agile, and customer-focused.
How to Choose the Right Tools for Advanced Analytics in 2025
Choosing the right tools for advanced data analytics is absolutely critical to uncover insights that drive company performance. Firms must select tools based on their goals, budget, and the complexity of the data, considering the rising diversity of platforms and technologies out there. Here is how to choose the most effective advanced analytics tools for your business:
- Understand Your Analytics Needs: Start by clearly defining your analytics goals. Are you focused on analyzing data in real time, segmenting clients, or predictive analysis? Understanding your objectives can help you select products that align better with your company needs.
- Scalability and Flexibility: Your tools should grow as your data does. Seek systems that can manage significant data and change with developing corporate needs. Scalability guarantees that your company may keep reaping the benefits of sophisticated analytics tools long-term.
- Integration with Existing Systems: The tool must, above all, fit your present systems and data sources perfectly. Search for cloud-based system compatibility and strong APIs in tools.
- Ease of Use: Choose technologies that are simple to use, particularly for team members who might not be data science professionals. While some tools might need a significant learning curve, others have drag-and-drop features that streamline challenging activities.
- Support for AI and Machine Learning: Often, advanced analytics includes artificial intelligence models and machine learning techniques. Select tools with built-in machine learning features if you want to include predictive models. Working with an artificial intelligence firm can assist in tailoring your analytics solutions to better fit business requirements.
Although it may seem daunting, choosing the right advanced analytics tools will assist you in succeeding with data-driven decision-making by informing your choices.
Real-World Applications & Business Use Cases
Advanced data analytics techniques have become indispensable across numerous businesses. Companies that use these tactics benefit by creating wiser, data-driven choices, thereby gaining a competitive advantage. Let's look at a few practical uses:
- E-Commerce & Retail: E-commerce makes excellent use of predictive analytics to forecast demand, streamline inventory, and customize consumer experiences. Businesses can customize product suggestions based on purchase history, consumer behavior, and market trends, boosting sales.
- Healthcare: In healthcare, advanced analytics tools such as sentiment analysis and time series analysis assist in analyzing patient health trends, forecasting disease outbreaks, and optimizing treatment methods. Predictive algorithms are increasingly being used by hospitals to forecast patient readmissions, hence cutting expenses and enhancing patient care.
- Finance & Banking: Financial institutions utilize cluster analysis and regression analysis to identify fraudulent activity, evaluate credit risk, and enhance client segmentation. Advanced analytics guarantees real-time decision-making, hence improving security as well as customer happiness.
- Manufacturing: Advanced data analysis methods drive predictive maintenance, which guarantees that machinery remains operational for longer, hence reducing downtime. Machine learning algorithms can predict equipment failure, saving firms significant maintenance expenditures.
A recent poll finds that 64% of companies using sophisticated analytics say their operational efficiency rises and partner with advanced data analytics solutions, which can help companies maximize operations, cut expenses, and enhance consumer experiences.
Why Businesses Need to Upgrade from Basic to Advanced Analysis
Basic analysis is often mere reporting and descriptive data analysis, and the insights gained are limited. But companies are beginning to realize they must move to advanced analytics to gain meaningful insights and make better-informed decisions.
- Uncover Concealed Insights: Basic analysis gives just an overview, whereas advanced methods yield deeper, actionable insights that standard approaches typically fail to discern.
- Forecast Future Trends: Advanced analytics lets businesses examine the past and predict the future. Predictive analytics and other techniques help businesses forecast consumer behavior, sales trends, and market moves ahead of time, increasing their competitiveness.
- Improve Efficiency & Cut Costs: Sophisticated data analytics techniques enhance processes by highlighting inefficiencies and suggesting alternatives. By means of operational workflows, inventory holdings, and staffing needs assessments, companies can save a considerable amount of expenses.
- Stay Competitive: Data-driven decision-making helps businesses to remain profitable by staying ahead of the competition, reacting more swiftly to market change, and identifying new business prospects. Advanced analytics delivers the speed required in the fast-paced corporate environment of today.
Actually, 72% of those businesses that implement advanced analytics cite a 15% business performance improvement. Want to capitalize on advanced data analytics methods? Contact our subject matter experts at Avidclan Technologies—a top data analytics company offering customized solutions for your business.
Conclusion
Advanced data analytics is now a need for companies trying to succeed in the data-driven world rather than a luxury. Advanced technologies such as regression analysis, sentiment analysis, and predictive analytics give businesses the knowledge they need to make educated decisions, from forecasting consumer behavior to streamlining supply networks.
Companies that progress from simple to sophisticated analytics have a competitive advantage and enhance operational efficiency, lower expenses, and increase general performance. Partnering with an experienced data analytics company guarantees you are maximizing your data while you keep investigating the outstanding possibilities of data. Embrace modern data analytics to unleash new growth prospects and position your firm for long-term success in 2025 and beyond.
FREQUENTLY ASKED QUESTIONS (FAQs)
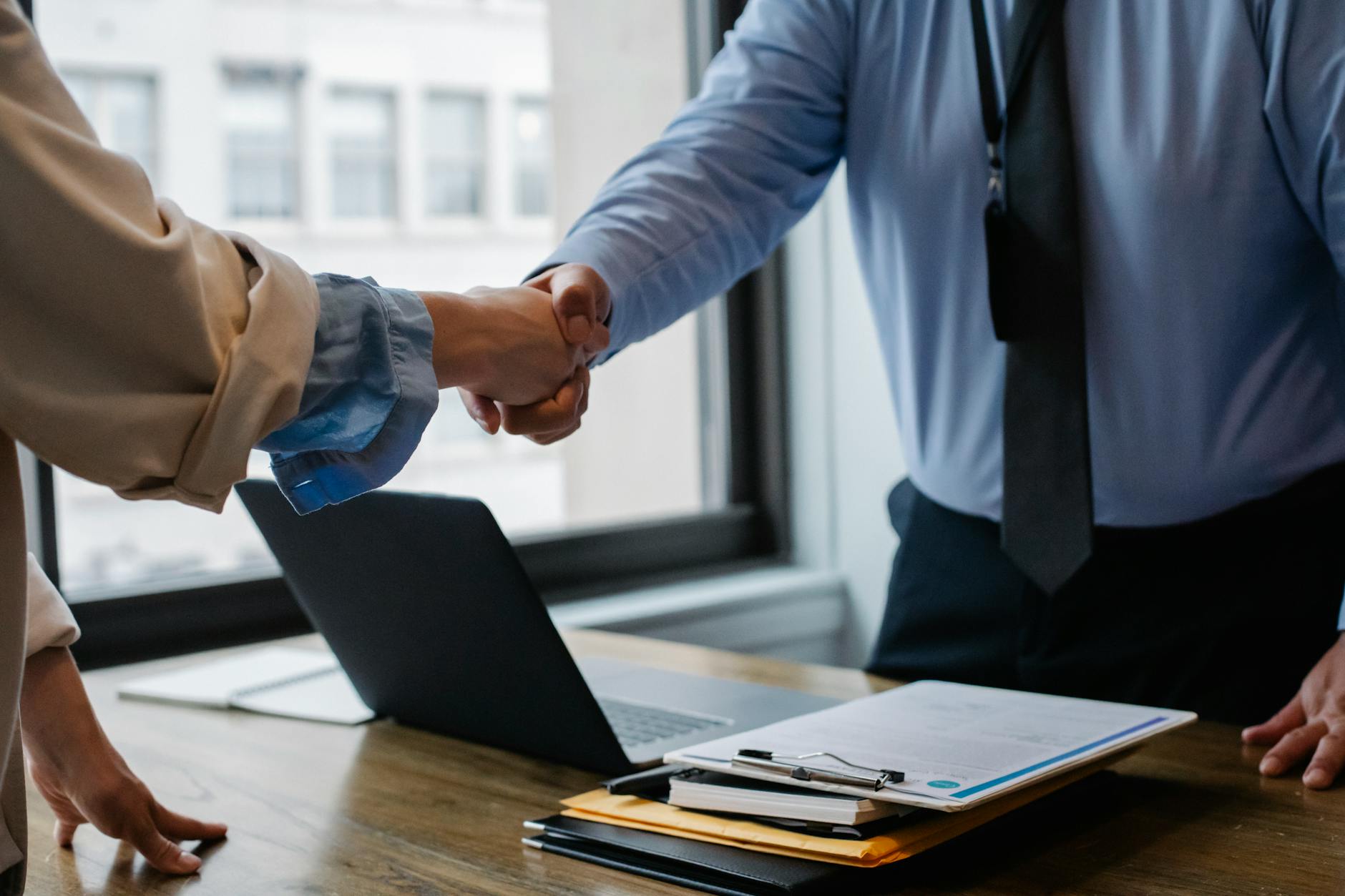